by Helen Morley, Learning Technologist, University of Sussex
How should we speak about the processes of Large Language Models and other AI? Language, as humans and other animals use it, is a voluntary and intentional tool which is used to aid interaction. Can we say the same for AI generated text?
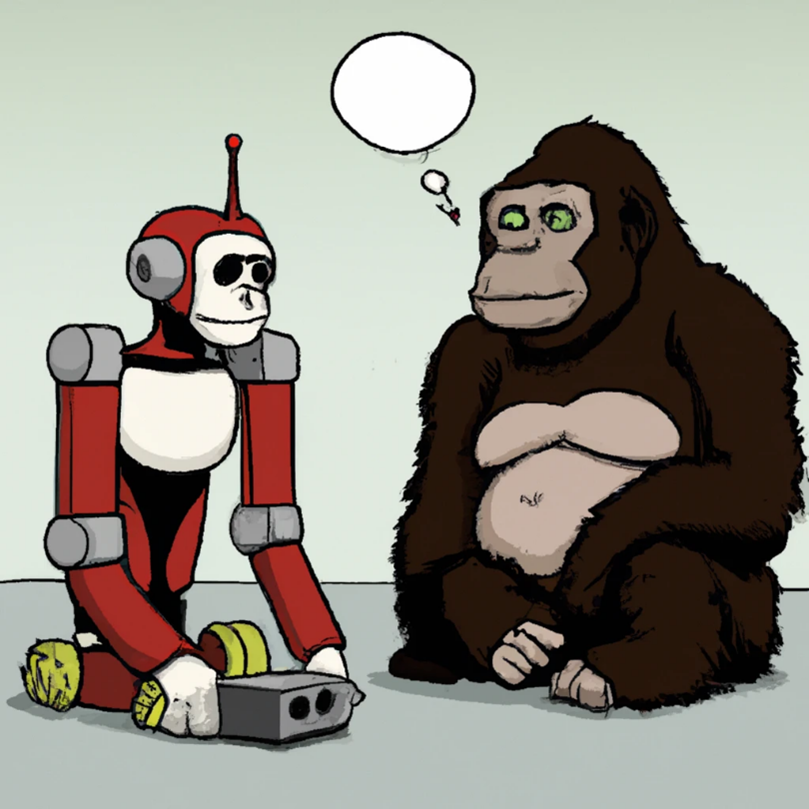
In November 2023, Professor Gilly Forrester delivered a lecture entitled “Hand to Mouth: The Language Puzzle” at the University of Sussex. I was excited to attend. It was a fascinating account of her studies into the correlation between anatomy and language skills, in particular how humans (and other great apes) demonstrate links between our manual dexterity and our communication with each other. Professor Forrester spoke about some of the neurobiology of language and the criteria some use to determine what language is. The lecture began with Professor Forrester asking us all how we would define language; for me, the answer is “a tool we use to help us understand what’s going on!”
That same month, I joined a webinar hosted by the American University in Cairo with Anna Mills as guest speaker. The topic was (as much of my 2023 also had been) “Artificial Intelligence in Education” with a particular focus on how AI can be used in writing classes. Mills’s observations, and those in the chat, turned at points to the phenomenon of AI generating false text. Words used to describe this included “fabrication” and the more popular “hallucination”. I have long been an advocate of the precise use of lexis and while I don’t particularly like either of these suggestions, I felt it necessary to explain why – for me – “fabrication” was not appropriate and to bring the conversation to readers of this blog to see if we can agree on a more suitable term. In other words, if we can make use of this language tool of ours to understand WHAT’S GOING ON?
I railed against “fabrication” because to fabricate something is to make it, and to do so with intention. Early uses of the word alluded to skill, and the Latin root refers to craftmanship and purpose. Today we use “fabricate” for the process of making something with a purpose; it is also used as something of a euphemism for dishonesty. Neither of these definitions are appropriate for describing AI programs generating inaccurate text: Large Language Models have no sense of purpose and they’re neither honest nor dishonest!
I railed against “fabrication” because to fabricate something is to make it, and to do so with intention.
I was thrilled to see Mills take this point to X (as @EnglishOER), where she asked for more ideas about what terms we could use to describe what the AI is doing in these situations. It is not sentient, conscious, creative, benevolent or malicious. It is not “making it up” any more than any other occasions when it strings words together into what we accept as a sentence. So what is it doing?
The TL;DR is that LLMs predict the next most likely word. They do this by “reading” existing texts and spotting the patterns. The sky is…, the dog goes…, my old man’s a…. It is this huge corpus of texts which is responsible for the biases we see in LLM output and for the erroneous accusations that some students’ work is AI-written when they’re actually just formulaic writers. The point is, ChatGPT does not think about what to output – it doesn’t think at all!
Not thinking, and not being competent to think, also rules out “hallucination” which I originally preferred to fabrication as at least hallucination is involuntary. It’s still not quite right and, in anthropomorphising AI, it risks confusing the matter further. When Mills put the question to her followers on X her criteria was: “the word shouldn’t imply conscious experience or intent”. The responses included: concoction (which was for a while her favourite); debris (too random and not representative of the coherent nature of LLM output); SLU which stood for “Statistically Likely Utterances” (dreamed up by Edward O’Neill with the enviable X handle of @learningtech); phantasma; and my contribution, “jibbering“.
As the year drew to a close, Mills did what so many of us spent 2023 doing: she turned to the tech. She gave ChatGPT itself the criteria, expanded to include that the term mustn’t imply intent or conscious experience; it must imply untruth/unreality, it should reflect patterns from training data but go beyond said data; the term must be accessible without having to be explained, be catchy and be memorable. I’ll let you decide if it’s disconcerting or not that ChatGPT output one of the best suggestions of them all. It called the product of its plausible, informed but false jibberings “data mirage”.
I’ll let you decide if it’s disconcerting or not that ChatGPT output one of the best suggestions of them all.
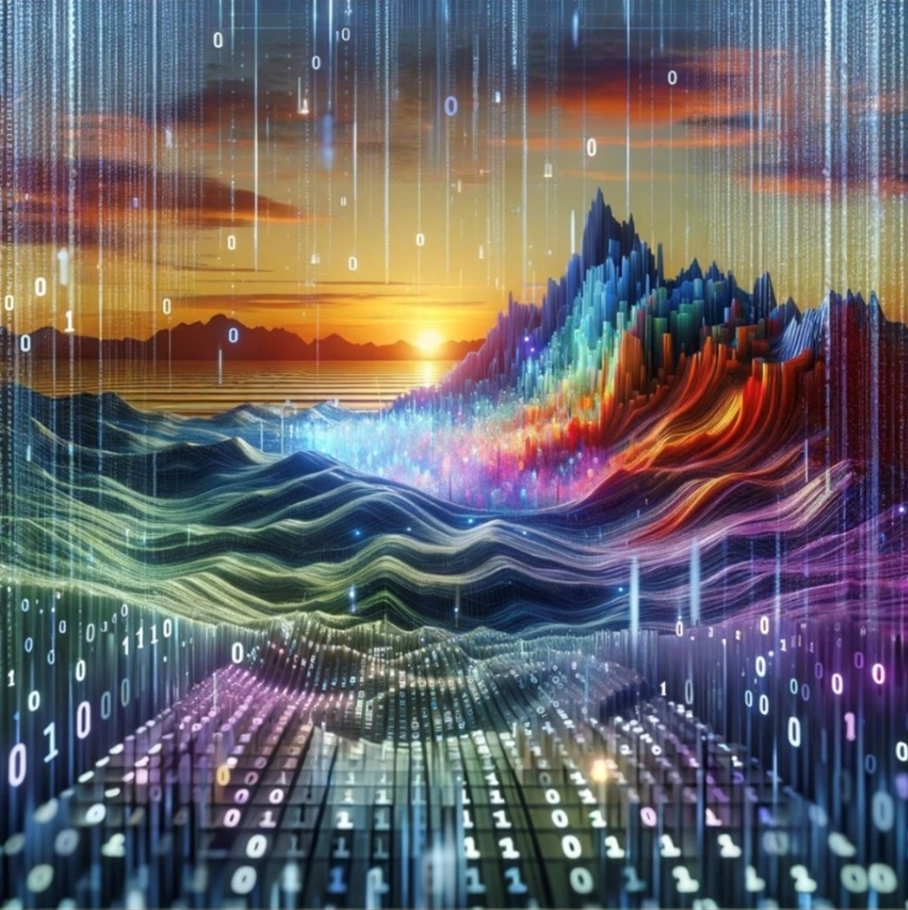
That’s it. Data Mirage. The data is real but what we experience is not. The unreal generation that we witness is not the fault or the design of anything else and it falls to us to determine whether what we are witnessing is to be trusted.
After all, we’re the Great Apes that are supposed to understand what’s going on!
More: Anna has compiled a list of suggestions she received here: https://bit.ly/HallucinationAlternatives
Watch Anna’s webinar and read about her work here:
https://learnhub.aucegypt.edu/digitaltoolkit/index.php/2023/10/30/generative-ai-activities-for-the-writing-language-classroom-anna-mills/
Leave a Reply